layers and lawyers, oh my / 2023-02-06
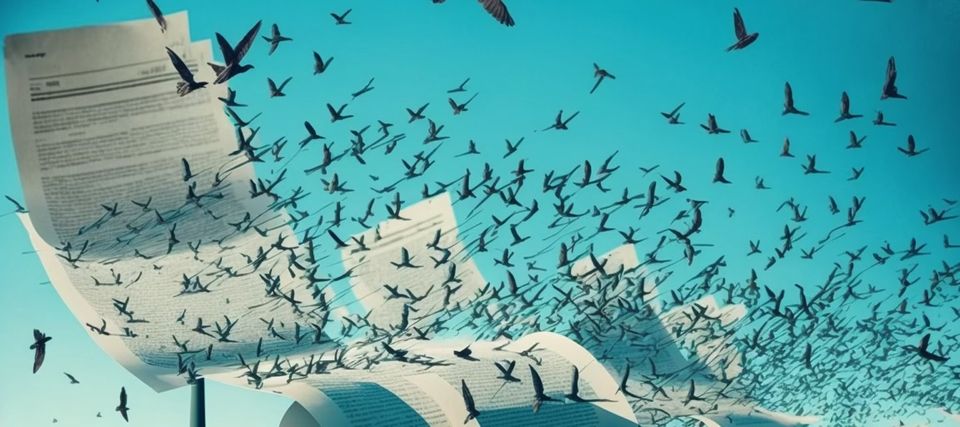
Have been sick, been “having a day job”, and…the world continues to spin.
ML bon mots (bon mlots?) of the week
- on training ML off a web corpus that will increasingly be some-percentage ML-generated: "If you liked Garbage In Garbage Out, you're going to love Garbage In Garbage Out And Then Back In Again."
- This:
It's only machine learning if the model comes with a manifest detailing the provenance of the training data.
— julia ferraioli (@juliaferraioli) February 6, 2023
Otherwise, it's just ✨sparkling✨ mystery bias recycling.
Governance of data?
A recurring theme of conversations in the past two weeks is that:
- open software has not done a very good job of standardizing governance (especially for community-led projects); and
- open data’s needs for governance are going to be very demanding relative to open software’s.
(1) is not inevitable: there’s plenty of demand for high-quality open software governance. Each of Apache, Linux Foundation, Eclipse, and (intriguingly) Open Collective have hundreds of projects. And (internally) they’re standardized. But that standardization is not well-communicated—in particular, even many experts in the area are surprised when I explain the implementation details of LF and OC, and we’re all still grappling with the implications of Eclipse’s move to Europe. So as building blocks, there's not a lot for others to use or "just drop in" the way we drop in license text files.
On (2), there’s a lot of experimentation in data and ML governance right now—here’s a good summary of some work on European data coops, for example, and there are several experiments going on around medical data, like U Chicago’s Nightingale and HIPPO. But none of these are standardizing quite yet, and people are beginning to ask hard questions and finding them lacking in practice.
I suspect early open software foundations would not have survived this sort of (warranted) skepticism. For example, Alex is 100% correct that metadata should be machine-readable, but most open software governance never mentions machine-readable license information—three decades in.
Because governance-oriented institutions are legally and technically complex, require ongoing work (and therefore ongoing funding), and (in some cases) may be more challenging in communities than corporations, this is an area where I would expect closed data/ML to have (short-term?) advantages over open(ish) data/ML communities. We don’t yet have a Creative Commons, but for governance organizations—it’ll be interesting to see if those evolve or not.
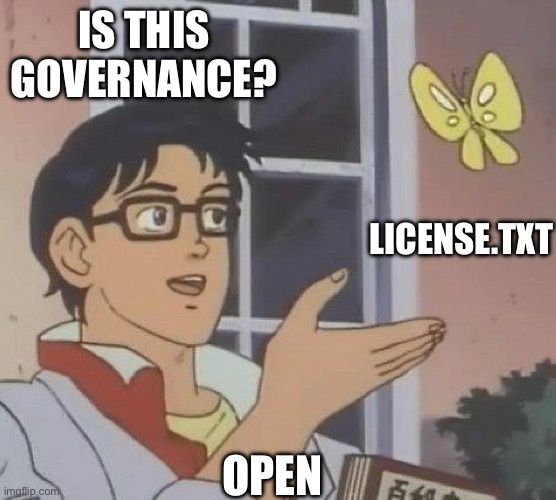
Attribution, cont.
I don’t know that this is open(ish) per se, but it feels like an important developing story about transparency and the allocation of power in this space—so sharing it.
We continue to see attempts to understand both whether something came from AI, and what sources were used to train that AI. Examples in the past few weeks include:
- OpenAI releasing a tool that will tell you if text is generated by ChatGPT… and then getting immediately slammed because its terrible false-positive rate will absolutely get innocent kids in trouble. They’ve also talked about their experiments in watermarking techniques, but it’s unclear if those are deployed in this new tool yet.
- Stable Attribution attempts to reverse-engineer Stable Diffusion to get at source images, but it took less than a day for people to find false positives. It’s also interesting to note that (to the extent the technique works) it is only because Stable Diffusion has (roughly) documented their training set; this approach would not be possible with the fully-proprietary Dall-E.
Remains to be seen if demands for this sort of transparency favor open(ish) models or not.
Models memorize training data, but...
I’ve heard said repeatedly, and probably said myself, that models do not “memorize” specific photos or text. Instead, they’re a collection of probabilities, and so they may generate images that look a lot like other images, but a specific image will not be memorized. This new paper (+explanatory thread from co-author) challenges that assumption, showing that some models do appear to contain “copied” images. This obviously has legal implications, and therefore implications for any open(ish) communities that want to implement ML.
A few important observations, most important last:
- Memorization is only sort of copying: The formal term for what is happening here is “memorization”, not “copying”: you can’t dissect the model and find a jpeg—anymore than your brain has a copy of the Mona Lisa inside. Rather, when the model is executed (often called “inference”) the probability matrix will output a nearly identical image. That may be a distinction without a difference for non-technical judges, but I suspect you’ll hear about it in the various pending litigations.
- Research only possible because of transparency: As with the attribution analyzer in the previous point, this research is only possible for models that have shared information about what they’re trained on. This research is, I think, important, but it would be damaging if it helps push models towards obfuscating their training sources.
- Duplication seems to matter: We know from some other contexts that doing de-duplication on a training set can be beneficial, both for speed of training and (potentially) for output quality. This may be another case of that—the paper seems to assume that duplicated images are those most likely to be memorized.
- One in a million: Initial tweeting about this paper made it sound like many images are “copied”, but the paper actually finds that 90 images are memorized out of 175 million images tested. I suspect that this is ultimately going to be seen as a pro-fair-use paper: a judge, faced with “this is transformative tech, but also there’s an extremely small chance that an extremely diligent adversarial search will find a copying” may lean towards a fair use finding.
This week in price/performance
This week in “low hanging fruit discovered in open ML”: 25% better performance in a small model by making a key constant a multiple of 64.
The most dramatic optimization to nanoGPT so far (~25% speedup) is to simply increase vocab size from 50257 to 50304 (nearest multiple of 64). This calculates added useless dimensions but goes down a different kernel path with much higher occupancy. Careful with your Powers of 2.
— Andrej Karpathy (@karpathy) February 3, 2023
Long thoughts: Lawyering up, but for who?
OpenAI and Stability AI have hired some of the best copyright litigators out there to defend themselves against the first wave of model lawsuits. (And literally as I write this, Getty has filed their opening salvo, backed by Weil Gotshal—an extremely high-end firm who represents many parts of the content industries.)
This partially answers a question I’ve pondered for a while—will the deep pockets of early ML defend themselves ferociously, or try to settle? Google spent nearly a decade aggressively defending itself which had, as a pleasant side-effect, the result of expanding fair use.
When I say "roughly a decade", I mean roughly 2001-2009. Google didn't stop fighting copyright cases after that date, but what changed in 2009? There, Google compromised, and tried to broker a deal to end the Google Book Search litigation that arguably would have expanded public access through Google but wouldn't have created new public access rights. I want to dig in a bit on why that compromise may have happened, and whether that can help us understand what will happen with Microsoft, OpenAI, and Stability AI.
Digression: why was Google fighting for fair use good for open(ish)?
There’s a lot of different dimensions to open, but most of them are enabled or enhanced when many actors can act without seeking permission from prior rights-holders. Want to talk about power? Fair use shifts that. Want to talk about innovation? Fair use distributes that. Etc., etc. So when Google created many pro-fair-use judicial precedents, that was pro-open.
Compromise cause #0: Cost
Google has definitely spent hundreds of millions, and plausibly over a billion dollars, on cases where fair use was a key part of the defense. (Disclosure: some of that went to me when I was working on Oracle-Google!)
There is no way to cut corners here; only the deepest pockets can fight these cases in a sustained, multi-year way. Microsoft has this capability; OpenAI might; Stability AI... not so much.
Compromise cause #1: Ideological drift
Early Google, especially in the legal department, hired a lot of pro-fair-use and pro-broad-innovation folks, compatible with “index the world’s knowledge” and “do no evil”. Those internal beliefs drove their early aggressive strategy, but as the ideological fervor weakened over time that made it easier to swallow negotiating a deal with publishers.
What do we know about the party’s internal beliefs now?
OpenAI has been pretty explicit that they consider broadly available AI an existential danger to humanity. So their long-term ideological commitments may run against expansive pro-public litigation, even if for pragmatic reasons they may fight hard for fair use today.
Modern-day Microsoft does open aggressively (and well) when it suits them. But their bottom line is obviously… the bottom line. So expect that, ideologically, they’ll do pro-open stuff if it is good for them—and likely not much further.
Stability AI is harder for me to read—if any readers have pointers, I’d welcome them!
Compromise cause #2: Counterparties and veto power
Quite simply, you can’t negotiate with the entire web. So even if early Google had wanted to compromise and go anti-fair-use, they couldn’t have—a settlement with one website owner would have opened them up to millions of lawsuits.
In contrast, because the Google Book Search litigation was a class action that claimed to represent (roughly) all US authors, Google could plausibly try to negotiate one deal that would cover all the scanned works. (Ultimately this approach failed, but it took several years to decide that.)
So far, the early ML cases look a lot more like the early web cases—if you accept the claims, the business probably goes away, because you can’t negotiate with the millions of dispersed data sources you need to do modern training. So OpenAI and Stability AI are likely to fight pretty aggressively. That said, I’m sure the deep pockets at OpenAI and Microsoft are thinking very creatively about how they could use their financial firepower to negotiate deals that would lock smaller players like Stability AI out. The Getty case (where there is one player, with a lot of high-quality content) may provide a test case for that. (The flip side may also occur: “we think this is fair use, but we don’t have the time to litigate that, so we’ll just cut Getty out altogether” is a plausible outcome as well.)
Compromise cause #3: Moat
For data obtained from the public, big parties like Google (2001-2009), Microsoft, and OpenAI often have strong reasons to fight for rules that increase public access. They know this increases the risk of competition, but if the other option is to not access the public data at all, then that’s a risk they have to take.
In Google Book Search, the dynamic was different—in theory anyone could have scanned several million books, but in practice that is expensive and complex. So Google didn’t need to fight as hard for public access.
Given the large size of modern training data sets, it seems unlikely that the core data sets will be amenable to this approach any time soon. But one could see well-funded companies avoiding public-benefiting litigation by striking deals for data sets used for many supplemental techniques we’ve discussed, like tuning or fact-checking.
Reading the litigation tea leaves?
I think that, so far, there are strong incentives for Microsoft and OpenAI to fight for legal precedents that will benefit open(ish) ML communities. But it’s certainly not a given, and I’ll continue following it.
Misc.
- Trusts in the wild: I’ve seen data trusts discussed as a replacement (or supplement) for data licensing for some time now, but this from the Suffolk Law Lab is the first I’ve seen them in the wild. Fascinatingly, if I understand it correctly, in this implementation every data user is a trustee, and every data donor a beneficiary—more extreme than I’d understood other past proposals. I still haven’t fully digested the implications, need to buy one of my trusts and estates friends a drink :)
- Telling fact from fiction in ML: @swyx is good on the problems of boosterism gone awry in ML.
- Bitcoin litigation is… relevant?: A UK court finding it plausible that Bitcoin developers might be liable for a breach of responsibilities. Has some potential ramifications for liabillity of any widely-distributed developer network.
- How do we regulate complex systems: This long article about banking regulations is very good on how governments regulate extremely complex systems. Suffice to say that the regulatory system is, itself, very complex—and ML regulation is likely to look like this in the future. In particular it may often operate indirectly on institutions rather than directly on specific technologies, which suggests governments will prefer regulable entities to diffuse communities.
- Trends in Python for ML: Even within open software, competition happens, and momentum and community matter a lot. It looks like Google’s TensorFlow is losing to PyTorch.
- Changing everything and nothing? I’ve written about how much I think ML might change. Eli Dourado makes an interesting related (counter?)point—it might change a lot of our day-to-day lives and still change very little about our economy.
- Slowly seeping into the “real world”, deepfakes in court edition: In the continued blurring of ML and the “real world”, Prof. Lilian Edwards asks what happens when someone deepfakes evidence in a court trial?
Personal notes
- I am flatted by how many people are reaching out to me about ML questions right now, and I’m also (literally) sick and (also literally) tired. So apologies if, despite the energy I’m trying to evince in the newsletter, I’m not getting back to you.
- I’ve started a re-read of one of Iain Banks’ Culture novels, in which AI plays an important role. I didn’t intend it to be a tie-in here, but… boy, they read differently in the current moment, both because the book’s Minds feel more plausible, and because the cultural gulf between those with ML and those without feels very real.
Member discussion