Deck the halls / 2023-12-06
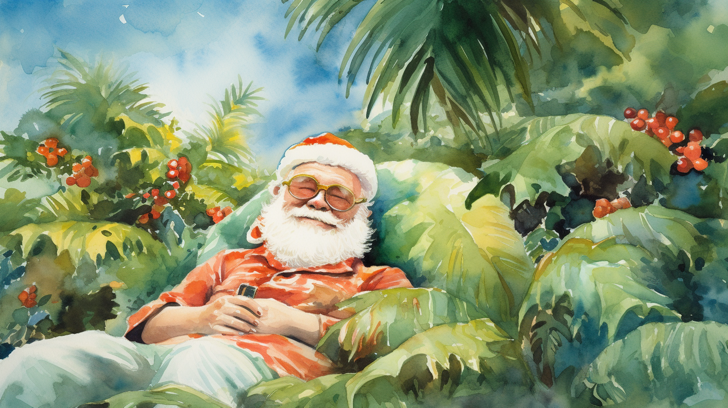
Intro
There’s been a lot in the past month. Can’t get it all (sorry, Executive Order) but dropping three long-simmering micro-essays—two up top, on OpenAI and Facebook, and one towards the end on how the switch from public licenses to 1:1 contracts might impact open.
Events
Next week: I’ll be speaking at AI.dev in San Jose on Dec. 12-13th on the layering of governance techniques in the search for a viable open AI. Drop me a note or comment (below) and let’s say hi!
Jobs
Micro-essay: Facebook, LLaMA, and Open
I've been doing other things during most of the kerfuffle around whether or not LLaMA2 is meaningfully "open source", as Facebook and Zuckerberg have repeatedly claimed. It clearly isn’t, as OSI explains here, and as I ranted about in video form here.
A few observations:
- Power matters: I've argued for a long time that we need to be generous when people misunderstand "open", and tolerant of near-open approaches. But it is one thing when young students trying to make the world a better place get confused, and another when someone who has been building on open source for nearly two decades—Mark Zuckerberg—does it. That’s true even if you give FB the benefit of the doubt on whether or not they are consciously openwashing—regardless of the motivation, the volume and power with which their message is projected increases the damage.
- Definition matters: Related to the previous point, it's one thing when a handful of activists challenge the definition of open source. It's another when a huge PR blitz (which is what this was, with articles in every major tech news outlet) mis-represents open source. The entire software industry relies on a shared, agreed-upon definition of open source to reduce developer and legal costs. If Facebook breaks that shared understanding by misrepresenting “open source” to the media and developer public, it's a big problem for all of us—including Facebook. Stephen O'Grady goes into this in a lot more detail. If you want to go even deeper, read up on open source as a "Schelling point" for the industry.
- Specificity matters: A lot of the most interesting attempts to do near-open or open(ish) tend to push very hard on one particular assumption of the current definition of open source, while embracing others. For example, the Ethical Source folks actively rejects the "no restrictions on use" plank of the Open Source Definition but embrace other community-enhancing parts of open. Facebook's terms (and approach) break the Open Source Definition in not just one, but several ways—they discriminate against specific users; they discriminate against specific uses; they do not provide even the slightest hint of the ability to rebuild the project's core artifact; and they produce uncertainty (reducing investment and innovation). If they’d picked one axis to violate, that would be one thing, but instead they break many, with the only real benefit for users and society of low cost—and mayyyybe certain types of amateur innovation.
- Clarity matters: I don't agree with Hashicorp's recent change away from open source, but at least they've got a fairly clear explanation of why they’re doing it. Facebook has made very little attempt to explain why they chose the restrictions they did, which makes PR blitz even worse. This could have been an intriguing opportunity for a real discussion of where open should go, if Facebook could have articulated why they're doing this. But they didn't—so instead it's left to the open source community to point out the confusion.
In short, if Facebook wants to have a real discussion about what open(ish) should look like in machine learning, I for one would welcome that—it's far from clear that "pure" open makes sense in this space. But the community should (and mostly has!) go hard after them for, instead, using their muscle to stomp on 25 years of industry investment and practice.
(And as I drafted this, another Meta driven announcement, this time with IBM, of an “open source” AI alliance: https://ai.meta.com/blog/ai-alliance/ No mention in there of... actual definitions of open. Also in recent open(?)washing(?): “Open”AI investing in open data.)
Micro-er-essay: corporate governance and alignment
Sadly I don’t have the time I’d like to go into the weeds of the OpenAI meltdown. But, one quick observation.
In the spirit of Ted Chiang on AI-as-management-consulting, I observe that the people who put together OpenAI simultaneously:
- believe they are capable of aligning new, complex, poorly understood AI incentives and techniques with human values; and also
- were demonstrably incapable of aligning age-old, simple, well-understood corporate incentives and techniques with human values (other than profit).
I am, to put it mildly, skeptical that anyone can do 1 if they can’t do 2. Corporate governance isn’t easy, but it’s a known, understood space, with repeatable strategies and playbooks. If you’re galloping so fast that you can’t get that right, the odds of you getting the unknown around AI right are low.
But open can’t get cocky—our record of alignment with any value other than “throw it on the web” isn’t great either. (Copyleft has arguably mostly worked by accident, not by careful legal design.) We will need to be critical and deliberate if we aren’t to have our own equivalent of the OpenAI fiasco.
If you want to get more in the weeds on OpenAI’s governance, this is good.
Values
In this section: what values have helped define open? are we seeing them in ML?
Improves public ability to understand (transparency-as-culture)
Actually transparent data sets continue to be important for journalists. This time, it is the Washington Post, explaining to the broader public how data sets can replicate American biases.
Increasingly I think that if I had to pick any one facet of traditional open to bring into AI, and give up all the rest, transparency for evaluation and explication would be the one I’d keep. Margaret Mitchell writes along similar lines, in a presentation prepared for the Senate.
Techniques
In this section: open software defined, and was defined by, new techniques in software development. What parallels are happening in ML?
Deep collaboration
There’s a lot of reasonable critiques to be made of GitHub’s methodology, but nevertheless I think it is worth noting that langchain (which I’ve written about previously) and a webui for Stable Diffusion are two of GitHub’s eight most active projects this year. Open collaboration around ML is very, very real.
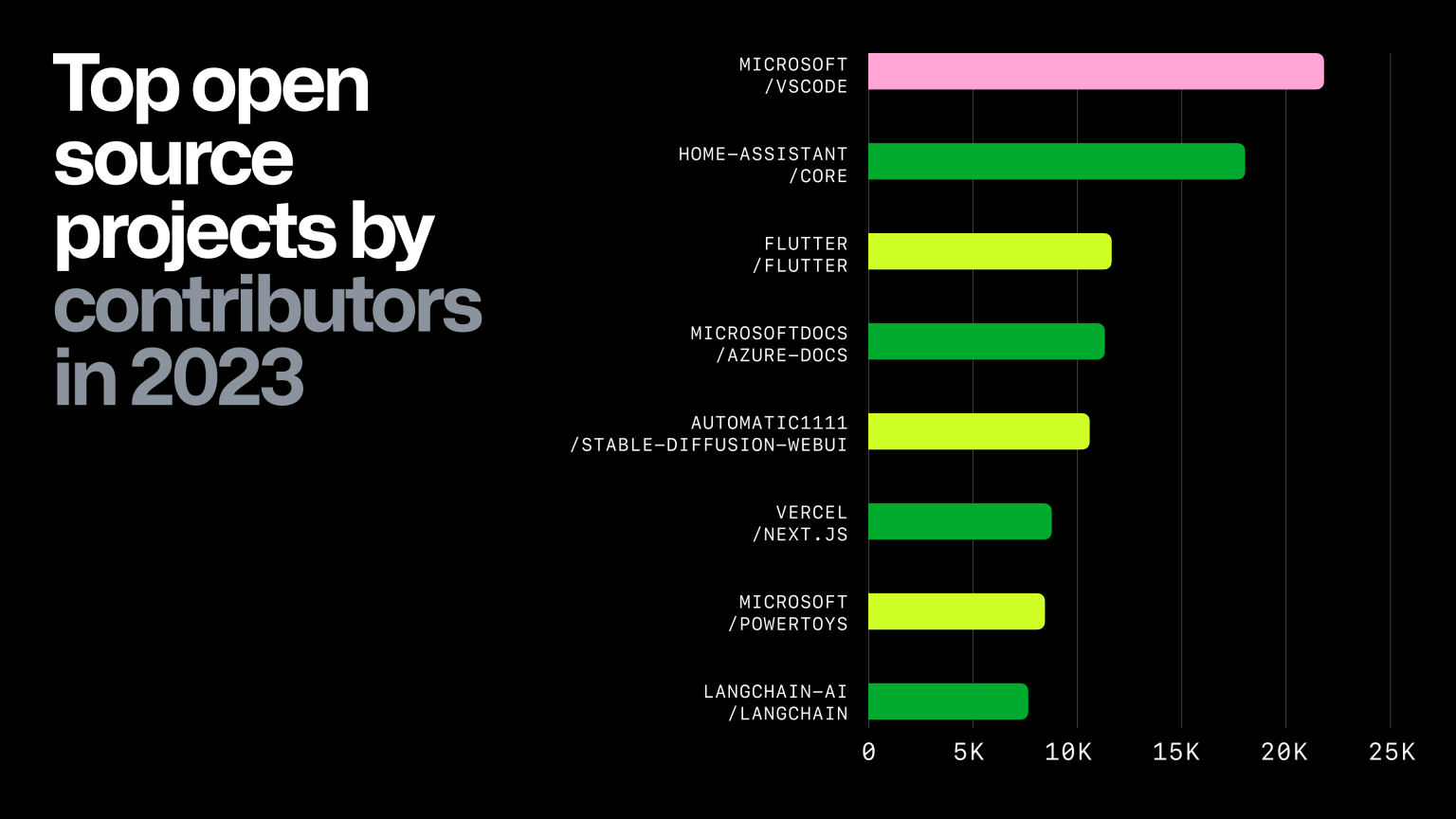
Model improvement
We keep getting performance wins, in part because openness enables broad investment in performance by parties other than Nvidia.
- faster inference on CPUs, not GPUs, via Intel
- very small model doing well on coding tasks
- the grossest C hack I’ve seen in some time—with good, cross-platform, inference performance (funded in part by Mozilla)
- Apple releases an open framework for high-performance ML on Apple Silicon
Joys
In this section: open is at its best when it is joyful. ML has both joy, and darker counter-currents—let’s discuss them.
Fundamental
I continue to see people, particularly in the old-school open community, saying AI is “useless, like bitcoin”. Here’s your counterexample of the week, where Deep Mind creates a catalog of stable crystals that may have a wide variety of industrial applications—one that they estimate might have taken 800 years to do with traditional approaches and investment. And they’re using robots to help build and test these in real life, as well. That’s genuinely awe-inspiring.
To put it another way: we need to be carefully skeptical of AI claims, but there really is a lot of baby in this bathwater. (Note that, like some other Deep Mind science projects, this work draws heavily on a previous generation of open science for training.)
Optimistic
More on one of my favorite topics, using very open ML to do translation on Wikipedia. The potential impact of this—if folks can still find Wikipedia in the blizzard of garbage!—is huge.
Pointless and fun anyway
Using ML to find tax fraud(?) through public art museum records(?!).
Changes
In this section: ML is going to change open—not just how we understand it, but how we practice it.
Regulated open, and moving from licenses to binding contracts
In March, I wrote that, increasingly, organizations may be legally prohibited from “true” open sharing for privacy, security, bias, product liability, and an increasing number of other reasons. This recent essay on the tensions between open data and other regulations in the UK power space goes in depth on a real-world example of this tension.
One way in which governments are pursuing such restrictions in a cross-border way are standardized contracts, which a sharer must force the recipient to execute before the thing is shared. At least in theory, this allows better enforcement: for example, you always know who the other party is (which enables data and model “recalls”), and contracts are less ambiguously enforceable than IP rights. This approach is most central in privacy, where the “standard contractual clauses” are over a decade old and commonly used to transfer data outside of the EU.
Unfortunately, it is hard to square these contractual requirements with traditional definitions of open sharing. I suspect this is why we heard this week that Facebook is considering a read-only Threads in the EU. If FB can’t execute a binding data agreement with each fediverse server, then FB can’t federate the posts of EU citizens to them. This should serve as a warning to other forms of open, federated sharing: mandatory contracts may break our norms.
Learning from privacy, responsible AI folks are thinking about how standardized contracts can be used to implement regulations and non-governmental restrictions. Here’s a new, long piece on it from The Global Partnership on Artificial Intelligence, that among other things touches on a variety of open licenses.
Unfortunately, the paper does not grapple deeply with key differences between open public licensing and executed and recorded standard contracts. The two share some legal similarities, but the mechanics limits who can participate in ways that are in deep tension with how open has traditionally been understood: minimal obligations for the sharing party, and low barriers to entry for recipients.
If standard contractual clauses become central to sharing in an AI age, I see two big questions for open:
- Are the standard/default clauses hostile to open values, or not? They obviously can't be open in the purist sense we've been used to, but there are many axes along which they could be more or less open - eg, they could be discriminatory or non-discriminatory (in the RAND sense); pro- or anti-competitive; simpler or more complex; high-cost, at-cost, or no-cost; etc. To put it another way: we can't use the OSI’s Open Source Definition to evaluate these agreements, because they will be non-OSD-compliant by law. But then how do we measure them? How do we influence them towards better ends even if they aren't OSD-open? (This has parallels to Simon Phipps' long efforts around standards licensing.)
- Are there entities that can execute those agreements on behalf of open projects? Agreeing to these sorts of terms will require, among other things, absorbing liability, controlling access, and providing technical infrastructure (like Turing Institute's data havens). I assume Linux Foundation will be going in this direction with their open data work; it'd be nice to see other hosting orgs also capable of offering this as a service to open communities.
I suspect that, in the near future, the business of “defining open” is going to look much more like these two questions than the traditional work of license analysis.
Misc.
- Kat Duffy has a good note on how improbable it is that the Executive Order on AI even exists. Worth a read to think through what this looks like from within DC.
- A lot of the challenges with responsibly and democratically governing algorithms are not new. This week it was France’s version of the EFF discovering badly discriminatory algorithms (trans), and it was the first I’d heard of this older but horrible British post office scandal, caused by buggy algorithms.
- I continue to believe that the least “sexy” but most important area of AI is evaluation: how do we know if AI is “good” at... well, anything? How do we know if tweaks make it better? worse? different? In this vein, some leading researchers have published a new benchmark, GAIA, featuring questions that humans answer at an 80% clip while ChatGPT answers at a < 20% clip. On the flip side, an interesting thread about responsible AI evaluation from... the Chinese government.
- I wrote a lot early this year about how one of the first places text generation was going to wreak havoc was on community moderators. Here’s an academic piece on how that is hitting at Reddit. TLDR: not great!
Closing note
This is old, but nevertheless: a hauntingly beautiful diagram of the vast human and technical complexity that goes into AI. Go ponder it for a few minutes.
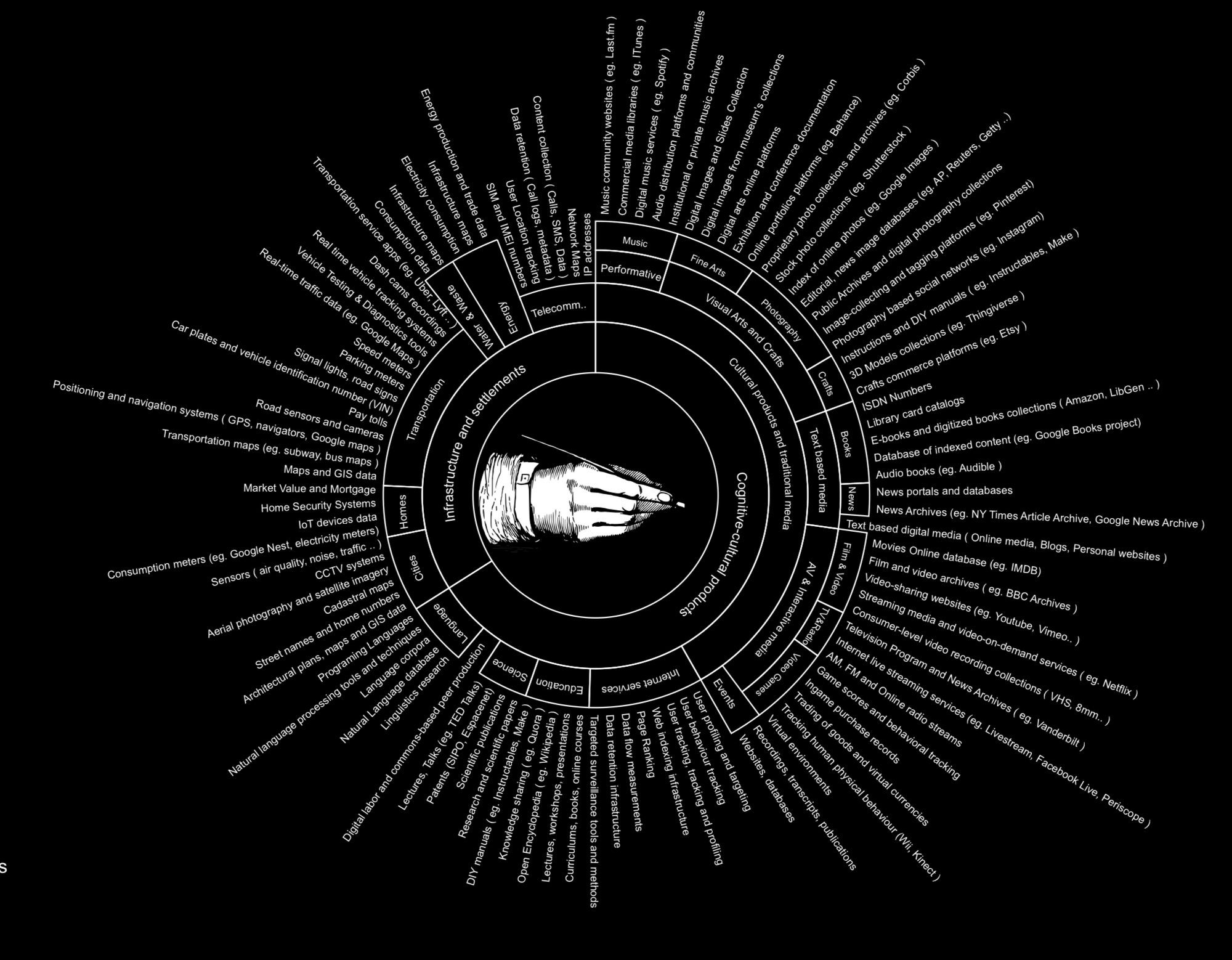
Our current software stacks are our cathedrals: intricate, beautiful art representing person-centuries of effort, binding together people from across wide regions. Also they will fall down a lot. The next generation will be even more so—powerful, beautiful, awe-inspiring, and (perhaps for a long time) terrifying if you think about them hard enough.
Member discussion