Blergh (viral and regulatory) / 2023-10-29
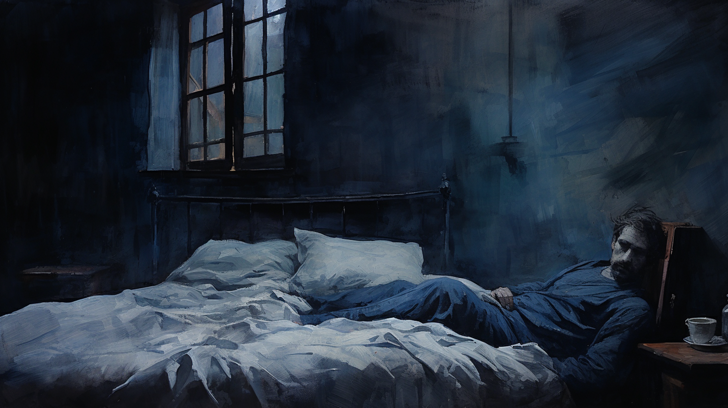
My introductory deep thoughts this week are “I went to a conference and got a virus, so far not covid”. So links, links is mostly what you get.
Events
If you’re in the UK, the AI Fringe is going on Oct. 30-Nov. 3 to complement the UK Government’s AI Safety Summit, and in particular to attempt to drag attention away from speculative future risks (what Yann LeCun of Meta has dubbed “EFRAID”) and towards present-day harms. Should be worth stopping in at.
If you can’t be there, the People’s Panel on AI is an interesting experiment in deliberative democracy that may be a fun way to follow along—essentially, trying to see the Fringe (and AI) through the eyes of a semi-randomly selected bunch of normal people.
News
The upcoming week is going to be all about regulation:
- In the EU, they were almost done with an AI Act and then the “foundation model” narrative bit hard, throwing that off track.
- The US Congress is unable to do anything, so instead we will get an executive order Monday morning. The scope of that will, apparently, be extremely broad; perhaps not explicitly banning open source large models, but certainly making them difficult to produce. For better or for worse, this is probably what the public wants.
- France has produced a less-sprawling attempt at new AI regulation that highlights how difficult even “simple” regulation is going to be. Among other things, because any given “source” has so little connection to outputs, the traditional-ish licensing approach of Spotify-like music subscriptions or “collection societies” will result in mere pennies to creators.
Values
In this section: what values have helped define open? are we seeing them in ML?
Lowers barriers to technical participation
Take all papers of this sort with a grain of salt, but here are some experiments showing that you can have LLMs ask users “what do you want” and get better outcomes than elaborate prompting. Doesn’t take much to imagine going from that to increased accessibility of common technologies.
Enables broad participation in governance
Tech workers are not used to thinking of themselves as “labor” in the activist sense, so my inclusion of an article on the terms of the Writers’ Guild contract may seem off. But this thoughtful piece goes into some good details on how they’ve thought about AI and its role in their collaboration. Critically, author Eryk Salvaggio says you can look at the deal through the lens of four values:
Authority negotiates who has the right to deploy AI in a project, and when.
Agency negotiates how AI is integrated into a workflow.
Disclosure refers to acknowledging the generative origins of any material.
Consent allows writers to negotiate terms for training generative systems on the material they make.
We could do worse than thinking these through in the context of building the tech as well.
Improves public ability to understand
A group of top researchers published the Foundation Model Transparency Index (FMTI), a very systematic attempt to understand how transparent major models are, covering 100 different facets of transparency. It got immediate media attention.
Its methodology also got pretty immediately dumped on. This critique points out that many of the listed factors are good/interesting, but don’t actually have anything to do with transparency or reproducibility—“the FMTI is comprehensive to the point of impracticality and goes beyond a reasonable set of disclosure requirements”. That article also points out that scoring points on “less impactful” indicators “might enable them to achieve higher scores while providing less actual transparency”.
And indeed that’s almost certainly what happened, because Llama (which has no transparency about its training sources, widely agreed to be the single most important form of transparency) scored ahead of Bloom—which is completely open about every step of its training. Eleuther.ai goes into great detail on these problems.
Besides going into how FMTI gets Bloom wrong, it also goes into how the entire framing of FMTI is somewhat problematic—particularly that many of the questions asked are really about hosted services or companies, not models. (One could, and should, ask transparency questions for both models and hosted services—but they’re different questions about different beasts, and FMTI apparently conflates them.) This last post is definitely worth a deep read if you really want to understand what transparency can, or should, mean in this moment.
Shifts power
- Conflicting reports earlier this month on whether Microsoft is finding AI profitable yet. I suspect that’s mostly an accounting question at this point, but still interesting that it is even in question for Copilot. (Put under power because the costs of all this have a significant impact on how power plays out!)
- Antitrust folks continue to talk about ML. Nothing particularly new in that piece but possibly useful if you’re catching up.
Techniques
In this section: open software defined, and was defined by, new techniques in software development. What parallels are happening in ML?
Explainers
Simon Willison has a great explainer on “embeddings”, a term that gets thrown around a lot but rarely defined. I learned a lot from this and recommend spending time with it if you’re trying to learn the tech better.
Model improvement
A fundamental question for regulators of ML, including (perhaps especially?) open ML, is the ultimate impact of the current model techniques. If they’re genuinely super-powerful, then extremely strict regulation (like nuclear weapons, or child pornography) may be appropriate. If they’re not, then more “lossy” regulation (like what we place on pirated music) may be appropriate.
Yann LeCun, a long-time leader in the space and Meta employee, has been increasingly publicly saying that the current generation of techniques simply can’t get there. Here’s a shorter version of the same argument from his twitter account.
One of LeCun’s key arguments is that the current techniques can’t plan, and a pair of papers came out this week that come to the same conclusion (Twitter thread).
Deep collaboration
Benedict Evans has an interesting essay on how ML might turn into products that is worth reading. He points to a variety of factors like whether the default mode for products will be thin wrapper around powerful model, or thick wrapper around lighter-weight model.
While he never mentions open, one underrated way that open software has impacted the world is that providing lots of well-defined technical building blocks has allowed more time to be spent on product design. What the industry begins to expect from ML will change both how open models are developed (do people even want open models?) and how other layers of tech are deployed, so this is worth keeping an eye on.
Transparency-as-technique
Open source has traditionally insisted on tying together the right to transparency and the right to modification, but that’s not a given—increasingly society will want, if possible, to have transparency into authenticity—perhaps not preventing modification, but at least tracking modification.
I’ve mentioned Adobe’s content authentication initiatives here before, and now we have a professional camera that builds in authentication tool chain. Tim Bray, camera geek and software guru, has more on the technical details of the C2PA. He concludes: “I’m in favor of anything that helps distinguish truth from lies”.
This won’t really take off until it is built into iPhone and Pixel, but still an interesting look at a potential, validated future.
Joys
In this section: open is at its best when it is joyful. ML has both joy, and darker counter-currents—let’s discuss them.
Once a Wikipedian, always a Wikipedian, so I’ll always be a sucker in this section for examples of Wikipedia using ML models to improve communication—here, understanding what language is being used (a surprisingly tricky task with traditional techniques!)
Similarly, I loved this: ex(?)-Meta folks built a tool that finds dubious citations on Wikipedia and recommends better ones, which experienced editors prefer >2:1 over the existing citations: Tweet, blog
Closing note
I look forward to being healthy again.
Member discussion