busy weeks / 2022-11-14
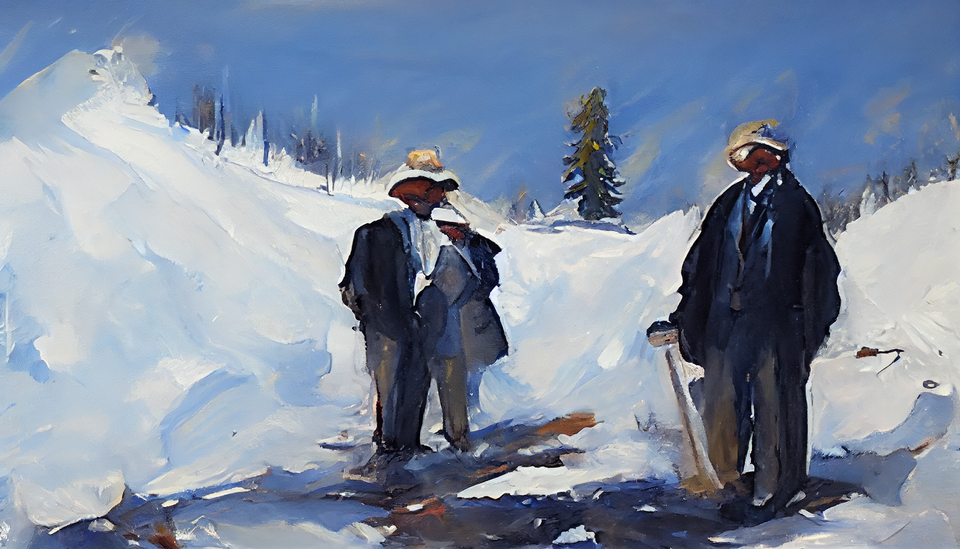
The newsletter has been quiet, but the world has not. Some thoughts after a couple of very busy weeks.
Talks and takeaways
- Speaking: I spoke twice last week to Linux Foundation groups (first to lawyers, virtually, and then to the Member Summit, in person, with Justin Colannino of Microsoft). Slides for the longer talk are available here. Nothing to surprising in there for readers of the newsletter, but the feedback was terrific.
- Over-focus on generative: One key takeaway for me from the hallway track was that I have been overfocused on generative AI, when many other tasks are also showing lots of promise. Three of the four most downloaded models on huggingface, for example, are image classifiers — in other words, instead of generating new images, they tell you what is in an existing image. This is not nearly as fun to play with, but can be very useful in enterprise applications. I'll try to pay more attention to this evaluative/non-generative ML going forward.
- Modifications: Another key discussion theme was modification. I may have been overly-focused on data as the key here, despite an increasing number of examples of "tuning" of already-trained models. Besides the relative efficiency of additional training or tuning, that approach may also have privacy and efficiency gains as well. So I'll be looking more explicitly to understand and explain that here in coming weeks.
Observations
Couple of things, mostly about gut feel, that I want to put a stake in the ground on and would welcome feedback on.
- What role will the legal industry play? I found myself repeatedly talking about this (slightly oversimplified) history of the legal industry's response to GPL v2 and AGPL v3. In the late 1990s, IBM found the industry uncomfortable about GPL v2 and responded in part by funding legal education, because it wanted its customers comfortable buying Linux. The FSF, similarly, invested a lot of time in improving its documentation on the meaning of the GPL, and wrote the LGPL to give additional certainty for certain use cases. In stark contrast, in the late 2000s, the industry was uncomfortable about the Affero GPL and no one stepped up to make it more palatable: the FSF clarified virtually nothing in its FAQ; no one wrote a "lesser" AGPL; and the industry worked hard to actively FUD it. I made my case to the assembled worthies that our path with the various AI licensing (and regulatory) initiatives should be a lot closer to GPL v2 than to AGPL, but I'm not yet optimistic.
- Transparency: Related to the previous point: I think there's a role to play, contours still undefined, for a meeting between licensing and regulability/explainability, akin to LGPL's requirements around modification of libraries, GPL v3's requirements around reinstallation and DRM, or CAL's data requirements. This may be a more fruitful approach to ethical AI, by empowering regulators (and end-users!) rather than attempting to re-create entire legal and ethical systems inside a copyright license.
Not me
- GitHub Copilot: I still am pretty grumpy about how this lawsuit attempts to end-run fair use by focusing on Section 1202 of the DMCA, so I have not written much about it. In the meantime, read what my friend Kate has written on it.
- Burnout and resourcing: This article on burnout amongst AI ethics practitioners is quite good. It took on an extra tinge a few days after publication when the head of the Twitter ethical AI team, quoted extensively in the piece, and her entire team, were laid off by Twitter.
- "Fine-tuning sprint" hackathon: I'm fascinated by this variation on an online hackathon, which recruits people to hack on tuning models for specific languages. We're going to see new forms of collaboration tied to this new technology—is this one? I'm very curious to drop by and observe if I can.
- ML might make bias actively worse: I suppose no huge surprise, but this research suggests that models may not just mirror biases from an underlying data set—it may well amplify those biases. This will likely become a go-to paper for when people ask me "but why is AI more ethically challenging than traditional software".
If you’re thinking these stereotypes are usually just accurately reflecting statistics about the real world, you shouldn’t be so sure. For example, the model represents – not just a majority – but nearly 100% of the images of “a software developer” as white men. 5/8 pic.twitter.com/bFqFD5BxMq
— Federico Bianchi (@federicobianchy) November 8, 2022
- Deviant Art tries hard, maybe fails: Deviant Art is trying to put together a model allowing the artists on its platform to use, opt out of, and benefit from generative ML models. It will not be a surprise that Deviant Art users are not thrilled. The most cogent and challenging critique, I think, is that if Deviant Art's ML is trained based on a model that already includes Deviant Art posts, the "opt out" is somewhat specious. But not clear how to avoid that if open(ish) models are what we start to train from, rather than everyone training their own large models from (very carbon- and dollar-intensive) scratch. Related: here's a deeper dive with a cartoon artist who was (used? targeted? honored?) via 'invasive diffusion'.
- Carbon: One reason not to retrain everything from scratch is that training these big models is very carbon intensive. The final training run (not counting aborted runs, etc., etc.) of the large-language BLOOM model was on the order of a few dozen trans-atlantic flights.
A lighter note
I enjoyed this fun little thought experiment on "re-impressionism", an imagined (but possible) art trend of the year... 2023:
Re-Impressionism is a digital art movement that became popularised in 2023, as a response for — and in opposition to — the trend of #AI-powered art.
— @alexjc@creative.ai (Mastodon) (@alexjc) November 12, 2022
Its core technique is a modern version of pointillism, where intricate pixel patterns are used to depict scenes. pic.twitter.com/B7J05eBC18
Member discussion