need ML to generate halloween puns / 2022-10-28
No big meta-themes this week. Enjoy Halloween :)
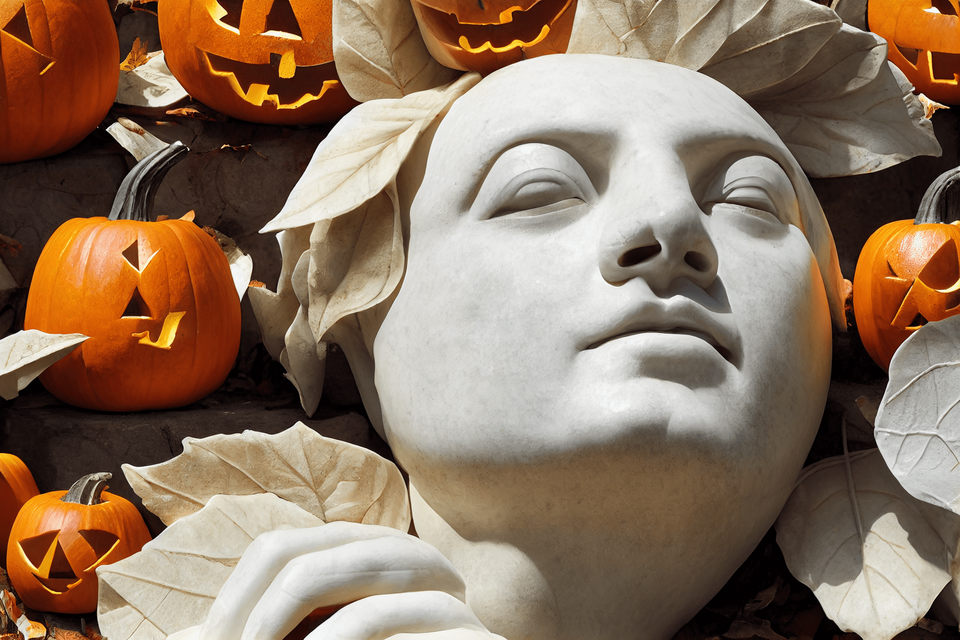
No big meta-themes this week. However, an observation: the industry seems to be coalescing quickly around "generative AI" as the name for "ML that makes creative things". I like it; harkens back (in a good way) to Prof. Jonathan Zittrain's conception of the "generative internet" c. 2006. Expect to see that term a lot going forward.
Open(ish) values
Lowering barriers to entry
- Open helps drive down costs, again: Another week, another set of anecdotes suggesting that a lot of the "ML is really expensive" discourse was, at least in part, driven by "ML has essentially unlimited budgets". Stable Diffusion's RAM usage can be down to 1Gb (from 48Gb a month and some ago), Intel is releasing 10-100x acceleration patches for commonly-used training toolkits, and training steps are getting cut in half for big language models (though still requiring academic supercomputers!) And here's a job posting to do more of this sort of optimization. The barrier to entry is coming wayyy down.
- Stable Diffusion continues to turn into an ecosystem: Here's a giant list of Stable Diffusion distributions, tools, etc. And here's the changes to it in just the past couple of weeks. Meta: I wish I had time to audit these for RAIL license compliance; it'd be a great way to understand license usage in the wild at a time when the licenses and other governance models are still adjustable.
Making systems legible
- What are we studying? I've talked here, optimistically, about the growing toolkit for analyzing models, to help us understand (and therefore improve and govern) them. But this thread points out that much of "AIthropology" is really study of OpenAI and OpenAI's choices, not study of "AI". The author points to study of more open models as a cure, but I have to wonder if the nature of training still means there's some black-box-ness. I will endeavor to be more clear here, going forward, between research and tools that are specific to a particular model, and research and tools that are more truly generic.
- Bias evaluation: Here's another addition to the tools for evaluating bias in large language models—essentially an (open data) test suite of bias-inducing prompts. There's going to be a lot of these; will be interesting to see if this one comes out on top since it is coming out of Huggingface.
Governance and ethics
- Weinberg on Copilot: Michael Weinberg, of many good things, has a worthwhile piece on the (potential) Copilot litigation. From the conclusion, something I increasingly wholeheartedly agree with: "Looking to copyright for solutions has the potential to stretch copyright law in strange directions, cause unexpected side effects, and misaddressing the thing you really care about."
Open(ish) techniques
Model improvement(?)
- Training models for specific styles: The first wave of copyright-infringement concerns in image-generating AIs were based on "styles" that the model learned somewhat 'organically' from captions in the training set. Now we've got a new technique that raises much more pointed questions, deliberately teaching Stable Diffusion about ("finetuning") specific styles. Meta: Is this open(ish)? I think yes, because "we don't need your permission to innovate" is, for better and for worse, a long-term correlate of traditional open.
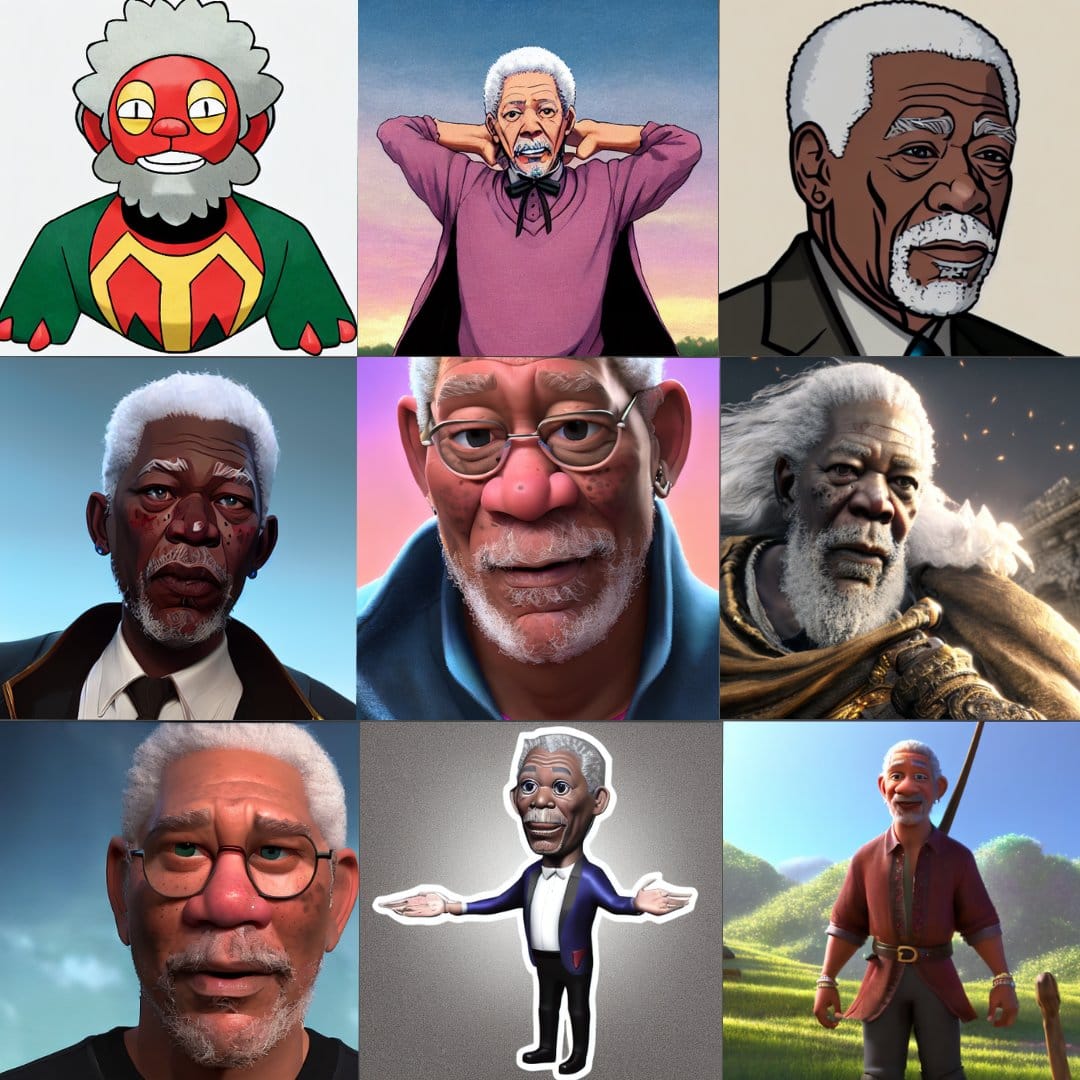
Instilling norms
- Norm of outcome-focus: It's very interesting to me, as a former QA guy, that the ML community treats quality of outcomes as worthy of academic research and publication, and that's taken seriously by practitioners! As best as I can tell, this is a side effect of ML's inherent unpredictability, and it feels like a very healthy norm to me—treat outcomes as importantly as you treat, say, performance or flexibility, and outcomes might actually improve. This thought brought to you by this paper on using prompts to improve "reliability"—including definitions of reliability in this context.
Changes
"creation engines"
At least this week, this section gets all the demos:
- Speak to a code-focused ML "like a product manager", get... actual changes to code? 👀
- Generate plot-heavy, coherent stories by training language models to do very human things like "rewriting" and "editing".
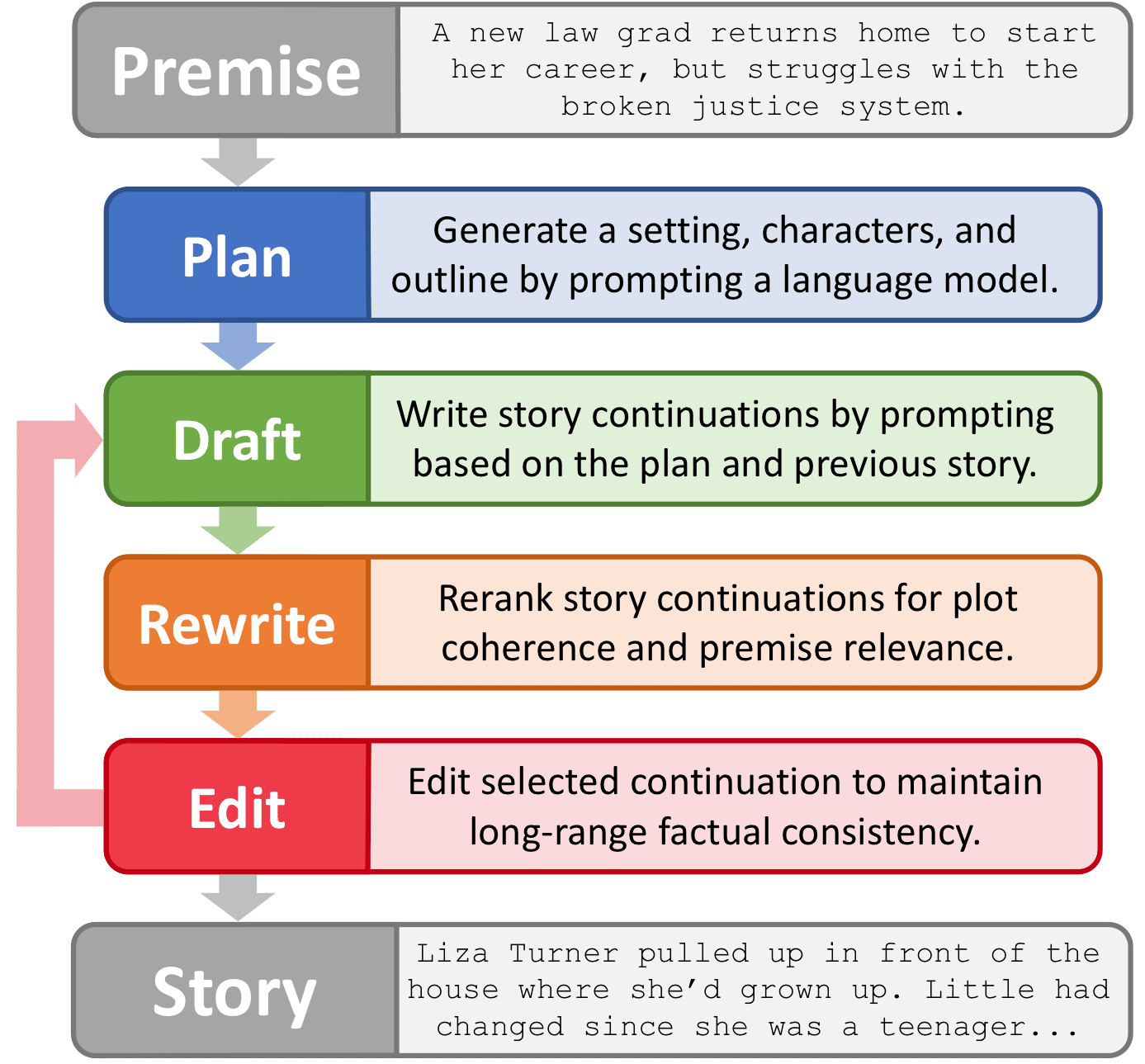
Meta/misc.
Some notes on what AI means for open from conversations and thinking I had this week.
- "Predictability": for users, a value of traditional open is predictability: they can read the license and figure out more or less what they can do, more or less quickly. I find this to be somewhat overrated (the scope of the GPL has never been super-predictable, but we got over that quickly when Linux became unavoidable) but it's still something I'll consider adding to my personal definition of open(ish). We're definitely in a period where this is not the case in open ML, but I suspect that's inevitable—and through a combination of ethical concerns and government regulation we may never get back to the simple, predictable world of traditional open. Nevertheless it's a factor for us to keep in mind.
- "Permissionless innovation": While thinking about the throwback phrase 'generative', and the variety of weird/exciting/problematic tools around Stable Diffusion, I keep coming back to the idea of "permissionless innovation", which is sometimes attributed to Adm. Grace Hopper (though perhaps not accurately)?
Thanks!
Thanks for continuing to join me on this ride :)
Member discussion